Support center +91 97257 89197
Generative AI developmentSeptember 24, 2024
AI-Powered Recommendations: Boosting Sales and Engagement in eCommerce
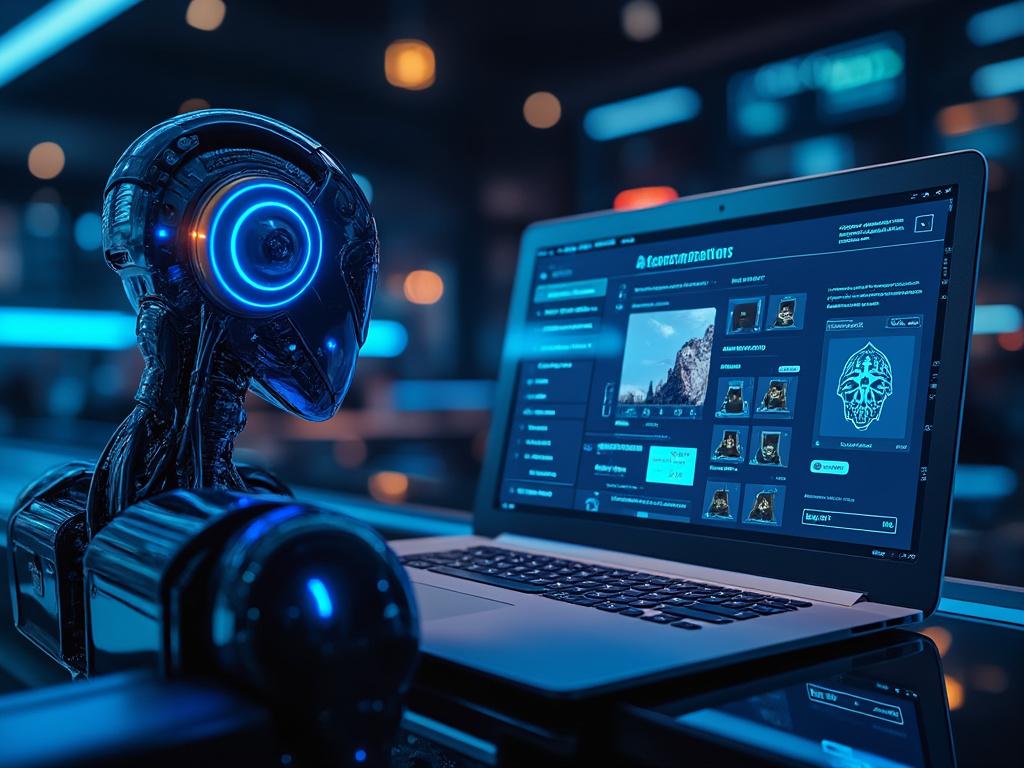
Introduction
The eCommerce landscape has evolved rapidly, with businesses constantly seeking ways to engage customers and drive sales. Among the many technologies reshaping the industry, AI-powered recommendations have emerged as a game-changer. By delivering personalized product suggestions, these systems enhance the shopping experience, foster customer loyalty, and maximize revenue potential.
This blog explores the science behind AI recommendations, their transformative benefits, real-world implementations, and how businesses of all sizes can harness this technology to stay ahead of the competition.
The Science Behind AI-Powered Recommendations
AI recommendations rely on machine learning models that analyze vast amounts of customer data to predict preferences. Here's a closer look at how they wor
- Collaborative Filtering
This method identifies patterns by analyzing user behavior, such as browsing and purchase history.
It groups users with similar tastes, enabling recommendations based on the actions of like-minded shoppers.
- Content-Based Filtering
Focuses on product attributes rather than user behavior.
For instance, if a customer buys a leather jacket, the algorithm might recommend other leather products.
- Hybrid Models
Combines collaborative and content-based filtering for more accurate recommendations.
Often used by platforms like Netflix and Amazon to provide a comprehensive user experience.
AI systems also employ natural language processing (NLP) to analyze textual data such as reviews, enabling a deeper understanding of customer sentiments. The integration of real-time decision-making allows businesses to present dynamic suggestions as customers browse.
Benefits of AI Recommendations in eCommerce
-
Enhanced Customer Experience
Personalization creates a sense of connection between the customer and the brand.
Tailored recommendations help shoppers find what they need more quickly, reducing decision fatigue.
-
Increased Sales Through Upselling and Cross-Selling
AI suggests complementary items (e.g., shoes for a purchased dress), encouraging customers to buy more.
Real-time insights can prompt offers like "Customers who bought this also bought..."
-
Improved Customer Retention
Personalized interactions foster loyalty, making customers more likely to return.
Consistent relevance boosts the overall customer lifetime value (CLV).
-
Efficient Inventory Management
AI not only tracks trends but also predicts demand, helping businesses stock the right products.
This reduces overstocking and understocking, minimizing waste and maximizing profit.
Implementing AI Recommendations in Your eCommerce Store
Getting started with AI-powered recommendations may seem daunting, but modern tools and platforms make it accessible. Here’s a step-by-step guide:
-
Integrate AI with Your Platform
Popular platforms like Shopify, WooCommerce, and Magento support third-party AI recommendation tools.
Plugins and APIs like AWS Personalize and Google Recommendations AI streamline the integration process.
-
Collect and Organize Data
Gather data such as purchase history, browsing habits, and user demographics.
Organize the data in a structured format for effective model training.
-
Train and Deploy Models
Use machine learning platforms to train recommendation algorithms based on your business data.
Deploy models to start delivering personalized suggestions in real-time.
-
Monitor and Optimize
Continuously analyze performance metrics like conversion rates and average order value (AOV).
Refine algorithms to adapt to changing user behaviors.
Case Studies of Success
-
Amazon
Amazon's recommendation engine generates 35% of its total revenue.
Through a mix of collaborative filtering, content-based methods, and AI-driven analytics, the platform delivers highly personalized suggestions that keep customers engaged.
-
Netflix
While primarily a media company, Netflix’s approach to personalization offers valuable lessons.
Its hybrid model provides tailored recommendations, which could inspire eCommerce strategies for cross-category recommendations.
-
Small Business Triumphs
A niche fashion retailer used AI to suggest outfit pairings based on customer preferences.
Within six months, they reported a 25% increase in average order value and a 15% improvement in repeat purchases.
Measuring the Impact of AI-Powered Recommendations
To maximize the value of AI recommendations, businesses must consistently track and measure their effectiveness. Key metrics include:
-
Conversion Rates
Tracks how often recommended products lead to purchases.
A higher conversion rate signifies that recommendations are resonating with customers.
-
Average Order Value (AOV)
Indicates the average spend per transaction.
AI often increases AOV by effectively upselling and cross-selling related products.
-
Click-Through Rates (CTR)
Measures how often customers click on recommended products.
High CTR reflects successful engagement with AI-driven suggestions.
-
Customer Retention Rate
Tracks repeat visits and purchases.
Improved retention rates demonstrate customer satisfaction with personalized experiences.
Tools for Tracking and Analytics
Platforms like Google Analytics, Mixpanel, and heatmap tools (e.g., Hotjar) help monitor user interactions with recommendations. AI tools like Dynamic Yield and Optimizely also provide in-depth insights into performance.
Challenges and Considerations
While AI-powered recommendations offer immense potential, businesses should be aware of certain challenges:
-
Data Privacy and Compliance
Ensuring compliance with regulations such as GDPR and CCPA is critical.
Implement transparent policies and obtain explicit user consent for data collection.
-
Algorithm Fairness and Bias
Biased algorithms can lead to skewed recommendations, alienating certain customer groups.
Regularly audit and test algorithms to maintain fairness and inclusivity.
-
Over-Personalization and Fatigue
Overloading customers with recommendations can lead to decision fatigue.
Maintain a balance by offering only a few well-targeted suggestions at a time.
-
Scalability
Smaller businesses may face challenges scaling AI systems as data volume grows.
Opt for solutions that are scalable and can handle increasing complexity.
Future Trends in AI Recommendations
The future of AI recommendations in eCommerce promises even greater sophistication and integration:
-
Generative AI for Advanced Personalization
Generative AI, like ChatGPT, can create tailored shopping experiences by crafting personalized product descriptions and emails.
This enhances customer engagement beyond just recommendations.
-
Augmented Reality (AR) and Virtual Try-Ons
AR tools integrated with AI provide personalized fitting and styling suggestions.
For example, AI can recommend clothing sizes based on user measurements or suggest complementary items in real-time during a virtual try-on.
-
Voice Assistants and IoT Integration
As smart devices and voice assistants become ubiquitous, AI recommendations will seamlessly integrate into these platforms.
Imagine receiving shopping suggestions directly through Alexa or Google Home while browsing an online store.
-
Sustainability-Driven Recommendations
AI can analyze user preferences for eco-friendly products and promote sustainable shopping choices.
This aligns with the growing consumer demand for ethical and green products.
Conclusion
AI-powered recommendations are revolutionizing eCommerce by delivering personalized, data-driven customer experiences. Businesses that adopt this technology stand to gain improved sales, higher customer retention, and efficient inventory management.
As the eCommerce landscape grows increasingly competitive, integrating AI tools is no longer optional—it’s essential. By leveraging the power of AI today, businesses can unlock new opportunities for growth and remain ahead of the curve.
TLDR
AI-powered recommendations revolutionize eCommerce by personalizing customer experiences, boosting sales through upselling and cross-selling, and improving customer retention. By leveraging machine learning tools, businesses can analyze user behavior, deliver tailored suggestions, and stay ahead in a competitive market.
FAQs
AI-powered recommendations use machine learning algorithms to analyze customer data and provide personalized product suggestions. These systems predict what a customer might like based on their browsing and purchase history, enhancing their shopping experience.
By suggesting products tailored to individual preferences, AI encourages higher purchase rates, increases average order value (AOV), and supports effective upselling and cross-selling strategies.
Popular tools include AWS Personalize, Google Recommendations AI, and platforms like Optimizely or Dynamic Yield. These tools integrate seamlessly with major eCommerce platforms like Shopify and WooCommerce.
Yes, many AI solutions are scalable and affordable. Small businesses can benefit from AI's ability to personalize customer interactions and drive engagement without requiring significant resources.
Commonly used data includes browsing history, purchase patterns, user demographics, and product attributes. This data helps AI systems train and optimize recommendation algorithms effectively.
Work with us