Support center +91 97257 89197
Generative AI developmentSeptember 10, 2024
AI-Driven Personalization in Advertising: Reaching the Right Audience at the Right Time
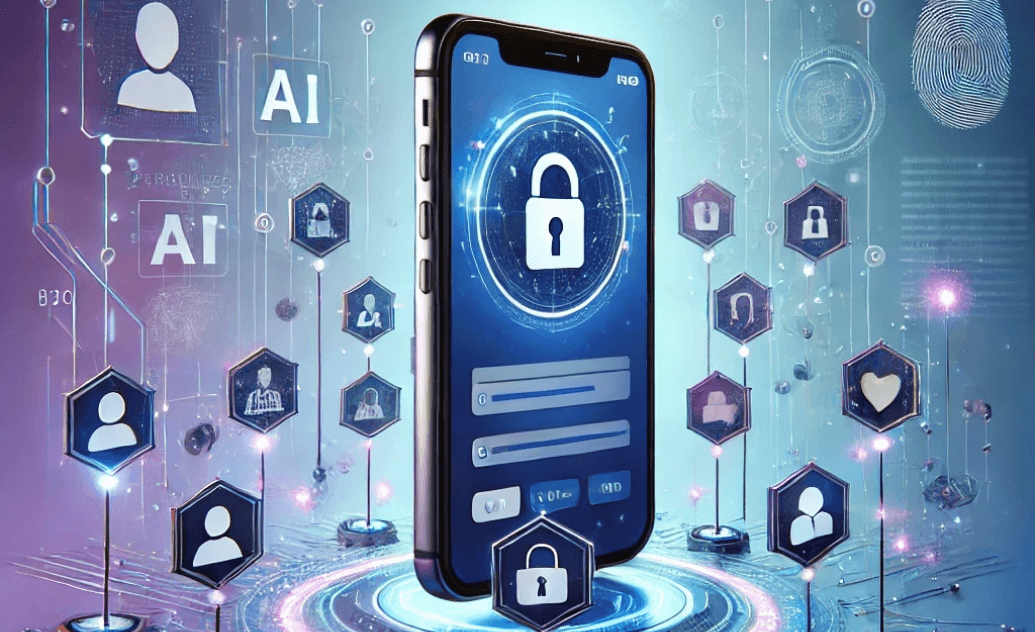
Introduction: The Evolution of Advertising in the Digital Age
As digital marketing has grown more advanced, the advertising landscape has shifted from broad, generalized campaigns to personalized, highly targeted strategies. Traditional advertising focused primarily on reaching a broad demographic with a one-size-fits-all approach. But as online data availability surged, a new opportunity emerged: personalization at scale.
With the rise of artificial intelligence (AI), brands now have tools to create real-time, personalized experiences that resonate with individual users based on their behaviors, preferences, and purchasing history. This AI-driven personalization enables advertisers to deliver the right message to the right audience at the most effective time. In this blog, we’ll explore how AI achieves this personalization, the technology behind it, and why it's quickly becoming a cornerstone in modern digital advertising.
Understanding AI-Driven Personalization: Key Concepts
What is AI-Driven Personalization?
AI-driven personalization in advertising refers to the use of artificial intelligence and machine learning (ML) to tailor advertisements to each viewer’s specific interests, behavior, and past interactions. Unlike conventional advertising that relies on static demographics, AI-driven personalization leverages real-time data and advanced analytics to build a dynamic and highly tailored advertising approach.
AI algorithms process vast amounts of data, recognizing patterns in user behavior to predict what will likely engage each individual. This process involves everything from basic segmentation—grouping users based on similarities—to complex predictive modeling that can anticipate a user’s next interaction with a brand.
Components of AI in Advertising
AI-driven personalization uses several core components and technologies:
- Machine Learning (ML): ML algorithms learn from data over time to improve ad targeting accuracy. For instance, they analyze how users engage with certain types of ads to better predict which ads to serve in the future.
- Natural Language Processing (NLP): NLP helps AI systems understand and respond to user intent through text and speech, which is crucial for personalized ad copy, chatbot interactions, and understanding sentiment in user-generated content.
- Predictive Analytics: This technique uses historical data to forecast future behavior. Predictive models can, for example, analyze purchasing history to recommend products a user is likely to buy next.
- Real-Time Data Processing: This allows for instant adjustments based on live data. For instance, if a user shows interest in a specific category, AI can modify which ads they see almost instantly, making the experience more engaging.
These components work in unison to create a cohesive, user-centric advertising approach that feels timely and relevant rather than intrusive or random.
How AI-Driven Personalization Works in Advertising
Data Collection and Analysis
The first step in AI-driven personalization is gathering and analyzing user data, which can come from multiple sources, including website behavior, app interactions, purchase history, social media engagement, and even location data. The data paints a detailed picture of each user’s preferences and behaviors.
Once collected, AI processes this information to identify key patterns. For example, a user who frequently browses outdoor gear websites might see targeted ads for related products. The more data AI can access, the better its predictions and personalization will be. However, this data collection must comply with privacy regulations like GDPR or CCPA, ensuring that users are aware of how their information is used.
Segmentation and Targeting
AI algorithms go beyond traditional segmentation methods by creating hyper-specific audience groups. While manual segmentation might break users into broad categories like “millennial shoppers” or “suburban families,” AI can create segments based on much finer details, such as “millennial shoppers interested in eco-friendly products with a budget over $50.” These segments allow for more precise ad targeting, increasing relevance and engagement.
Real-time adjustments are another valuable component of AI segmentation. For instance, if a user browses a travel website and searches for flights to Paris, they might immediately start seeing ads for hotels in Paris, travel insurance, or local attractions. This type of rapid-response targeting ensures the user receives ads that align with their current interests.
Real-Time Bidding and Ad Delivery
One of the most innovative applications of AI in advertising is real-time bidding (RTB), a process where AI participates in automated auctions to place ads in optimal spaces. When a user clicks on a webpage, an auction determines which ad they’ll see, and AI-driven algorithms analyze data to determine if the user fits the target profile for a given ad. If so, AI bids in real time, often within milliseconds, to display the ad.
This process, known as programmatic advertising, allows brands to optimize ad spend, ensuring that ads only appear for relevant audiences, minimizing wasted impressions and boosting ROI. By automating the process, AI also removes the need for manual ad placement, making the advertising experience seamless, cost-effective, and data-driven.
Benefits of AI-Driven Personalization in Advertising
Increased Ad Relevance
Personalized ads based on individual user preferences make advertisements feel more relevant and useful. Instead of a generic ad for all viewers, a personalized approach lets advertisers tailor messages to suit each person’s specific interests. This relevance makes users more likely to engage with ads, whether by clicking, learning more, or even making a purchase.
Improved Conversion Rates
The more relevant an ad is to a user, the higher the chance they’ll respond positively. AI-driven personalization often leads to improved conversion rates, as users receive ads that closely align with their interests and needs. Conversion-friendly ads save brands time and budget while delivering a smoother, more enjoyable experience to the consumer.
Optimized Ad Spend and ROI
AI-driven personalization doesn’t just improve targeting; it also optimizes ad budgets by ensuring each dollar spent goes toward reaching the right audience. Traditional advertising methods often involve broad targeting, which can lead to wasted impressions and lower returns. With AI’s ability to focus ad spend on users with high engagement potential, brands can achieve a higher return on investment (ROI). Programmatic advertising and real-time bidding (RTB) further enhance this by automating ad placements and minimizing unnecessary ad costs.
Enhanced Customer Loyalty
A more personalized advertising approach can also lead to greater customer loyalty. When users see ads that resonate with their preferences and interests, they’re more likely to engage with the brand and form a positive association. Over time, these personalized interactions foster brand loyalty by building trust and enhancing the user experience. Brands that deliver tailored experiences stand out to consumers, making them more likely to return for future purchases.
Examples of AI-Driven Personalization in Action
Case Studies in Different Industries
-
Retail: Online retailers like Amazon leverage AI to create personalized shopping experiences by recommending products based on past purchases and browsing history. This approach not only helps boost sales but also ensures customers discover products they’re genuinely interested in.
-
Streaming Services: Platforms like Netflix and Spotify use AI to provide personalized recommendations based on viewing or listening history. This keeps users engaged, leading to longer viewing or listening sessions and reduced churn.
-
Travel Industry: Travel sites such as Expedia and Airbnb utilize AI to suggest personalized deals, destinations, and accommodations based on a user’s previous travel habits, interests, and budget preferences.
Breakthroughs from Leading Brands
Brands like Facebook, Google, and Amazon are at the forefront of AI-driven personalization. Facebook, for instance, leverages AI to deliver hyper-targeted ads by analyzing user data across interactions, likes, shares, and comments. Google uses machine learning in its Google Ads platform to enhance targeting accuracy, showing ads based on users’ search queries, browsing history, and even location data. These platforms have set new standards for personalization, demonstrating the significant impact that AI can have on engagement and conversion.
Challenges in AI-Driven Personalization
Privacy and Data Security
A primary concern with AI-driven personalization is privacy. Since AI requires large datasets to create tailored experiences, user data collection and storage have raised questions about privacy and security. Regulatory frameworks such as the GDPR in Europe and CCPA in California mandate that users should have control over their data and that companies must be transparent about data usage.
Handling data securely, obtaining consent, and respecting user preferences are essential steps in addressing privacy concerns. Brands failing to comply with privacy laws not only risk fines but also damage their reputation, so a strong privacy strategy is key to responsible personalization.
Avoiding Over-Personalization
Personalization can backfire when it crosses the line into over-personalization. Users may feel uncomfortable or even invaded if they perceive ads as too intrusive or overly specific. A fine line exists between relevance and discomfort; if crossed, it can lead to a loss of trust. AI algorithms should be calibrated carefully to avoid displaying ads that feel overly intrusive.
Dependence on Data Quality
The effectiveness of AI in personalization depends on the quality of data. Inaccurate, outdated, or biased data can lead to poor personalization results, where users receive ads that aren’t relevant or reflect unintended biases. High-quality, up-to-date data is essential to effective personalization. This challenge also highlights the importance of ongoing data cleansing and validation to ensure accurate results.
Bias in AI Models
AI models can inadvertently carry biases if the data used to train them is skewed or incomplete. For example, if an AI model is trained on a dataset that over-represents a certain demographic, the personalized ads might fail to resonate with other groups. Regular monitoring and updating of AI algorithms, along with a commitment to data diversity, are essential to reducing bias in AI-driven personalization.
Best Practices for Implementing AI-Driven Personalization
Ensure Data Privacy and Transparency
It’s crucial for brands to handle user data responsibly. Transparent communication about how data is collected, stored, and used builds trust with users. Many brands now include clear privacy policies and consent options, ensuring that users understand how their data is being used for personalization.
Balance Personalization and Privacy
Finding the right balance between personalization and privacy can be challenging but is essential for user comfort. Brands can experiment with personalization levels to gauge user reactions, carefully tailoring ads without encroaching on personal boundaries.
Iterative Testing and Learning
AI-driven advertising is not a one-time setup but rather a continuous process. Brands should frequently test and refine their personalization strategies. A/B testing is especially valuable in personalization, as it allows brands to test various ad approaches to see which resonates best with users.
Monitoring and Adapting to Feedback
User feedback is an invaluable resource for refining AI-driven personalization. Brands should monitor user responses, click-through rates, and engagement metrics to identify trends and adapt their approach. An agile strategy enables brands to stay responsive to user needs and preferences, maximizing the impact of personalization.
The Future of AI-Driven Personalization in Advertising
Emerging Technologies and Future Trends
The future of AI-driven personalization holds exciting possibilities. Technologies such as augmented reality (AR) and virtual reality (VR) will likely play a larger role, allowing users to interact with products in immersive ways tailored to their preferences. Voice search is also becoming a powerful data source for AI, enabling even deeper personalization based on user speech patterns, questions, and preferences.
The Role of Predictive and Anticipatory Advertising
AI is evolving from reactive personalization to predictive and anticipatory advertising, where algorithms can predict what users might want or need before they even search for it. By analyzing patterns in user data, AI could soon deliver ads for products or services that match upcoming user needs, providing a seamless and proactive experience.
Potential Regulatory Changes
As AI personalization grows, so too does regulatory scrutiny. Governments worldwide are increasingly focused on protecting consumer data, with regulations that could influence how brands use AI in advertising. Staying updated with regulatory changes will be essential for brands to maintain compliance while leveraging AI for personalization.
Conclusion: Embracing AI-Driven Personalization Responsibly
AI-driven personalization represents a powerful tool for advertisers, enabling them to reach the right audience at the right time with tailored messages that boost engagement and conversions. However, as brands increasingly rely on AI, they must also prioritize data privacy, transparency, and ethics. By embracing responsible practices, brands can harness the full potential of AI while building trust and loyalty with users. The future of advertising lies in AI’s ability to blend technological precision with a genuine understanding of user needs—a balance that, when struck, can elevate user experiences and create lasting customer relationships.
TLDR
"AI-driven personalization in advertising transforms audience engagement by leveraging user data to deliver targeted ads in real time. This blog explores how AI optimizes ad relevance, improves conversion rates, and enhances customer loyalty while addressing the challenges of data privacy and potential over-personalization."
FAQs
AI-driven personalization in advertising is a process where artificial intelligence uses data like user behavior, interests, and preferences to customize advertisements for each viewer. This personalized approach helps brands deliver more relevant ads, resulting in higher engagement and conversions.
AI gathers data from multiple sources, including browsing history and past purchases, to identify user segments. By analyzing this data, AI can identify potential customers and predict their preferences, delivering ads tailored to each person’s interests and behavior.
Personalized advertising increases engagement by showing users relevant ads, improves conversion rates, optimizes ad spending, and can enhance customer loyalty by making users feel understood and valued. The result is often better ROI on marketing campaigns.
Yes, data privacy is a significant concern in AI-driven advertising. Since AI relies on user data to personalize ads, brands need to be transparent about data usage and comply with privacy laws. Ensuring secure data handling and respecting user consent are critical to ethical personalization.
Examples include streaming services recommending shows based on past viewing, e-commerce platforms suggesting products users may like, and travel sites tailoring offers based on previous destinations. These examples demonstrate AI’s ability to create personalized experiences across industries.
Work with us