Support center +91 97257 89197
Generative AI developmentSeptember 17, 2024
AI-Driven Predictive Maintenance: Keeping Manufacturing Operations Running Smoothly
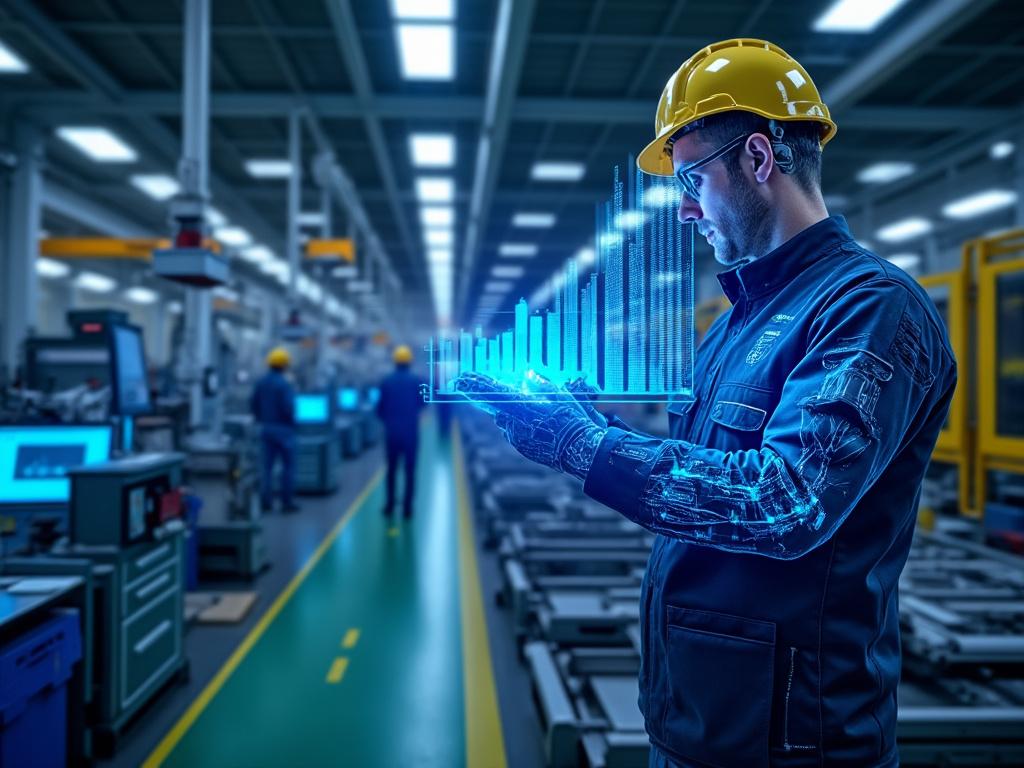
Introduction
In today’s fast-paced manufacturing environment, ensuring the continuous operation of machinery is critical to meeting production targets and maintaining profitability. Traditional maintenance methods, such as reactive (fix it when it breaks) or preventive (scheduled servicing), often fall short in minimizing downtime or cutting costs.
Enter AI-driven predictive maintenance—a game-changing approach that uses data, analytics, and machine learning to predict equipment failures before they occur. By addressing potential issues proactively, manufacturers can reduce downtime, lower costs, and improve efficiency. In this blog, we’ll explore the concept of predictive maintenance, how AI enables it, and the transformative impact it has on manufacturing operations.
What is Predictive Maintenance?
Predictive maintenance is a data-driven approach that identifies the likelihood of equipment failure based on its real-time condition. Unlike traditional methods, it relies on actual equipment performance rather than fixed schedules or post-failure fixes.
Key Differences: Traditional vs. Predictive Maintenance
Predictive maintenance is powered by advanced technologies such as the Internet of Things (IoT) sensors, big data analytics, and artificial intelligence (AI).
Key Technologies Powering Predictive Maintenance
- IoT Sensors: Collect real-time data on temperature, vibration, pressure, and other parameters.
- Data Analytics: Process historical and live data to identify anomalies.
- Machine Learning: Enable pattern recognition and predictive insights for better decision-making.
Role of AI in Predictive Maintenance
AI is the driving force behind the accuracy and efficiency of predictive maintenance. Here’s how it contributes:
Machine Learning (ML) Algorithms
Machine learning identifies patterns in equipment behavior by analyzing historical data. These patterns help in predicting when a part is likely to fail, enabling timely intervention.
Natural Language Processing (NLP)
NLP extracts insights from maintenance logs, work orders, and manuals, offering recommendations to technicians. For example, it might flag recurring issues or suggest optimal solutions based on historical data.
Computer Vision
Using cameras and AI, computer vision monitors equipment for visible wear and tear, such as cracks or misalignments, in real-time.
How Predictive Maintenance Works
- Data Collection: IoT sensors installed on machines gather data like temperature, vibration, and pressure.
- Data Processing: This data is analyzed using AI algorithms to detect anomalies and predict failures.
- Insights Generation: The AI system provides actionable insights, such as which part of the equipment needs servicing and when.
- Proactive Action: Maintenance teams use these insights to schedule repairs, ensuring minimal disruption.
Example Workflow
- Data from Sensors: Temperature sensor detects overheating.
- AI Analysis: Machine learning identifies a pattern suggesting motor wear.
- Notification: AI sends an alert to the maintenance team.
- Action: Technicians replace the motor, avoiding a costly breakdown.
Benefits of AI-Driven Predictive Maintenance
1. Cost Savings
Unplanned downtime can cost manufacturers thousands of dollars per minute. Predictive maintenance minimizes these costs by addressing potential failures before they escalate.
2. Improved Efficiency
Proactive repairs mean fewer disruptions, allowing the production line to operate smoothly.
3. Extended Equipment Lifespan
Regular, condition-based maintenance ensures machinery operates within its optimal parameters, reducing wear and tear.
4. Enhanced Safety
AI-driven maintenance helps identify hazardous conditions before they lead to accidents, ensuring worker safety and regulatory compliance.
5. Sustainability
By preventing equipment failure, predictive maintenance reduces waste and energy consumption, contributing to sustainable operations.
Real-World Applications
Predictive maintenance is transforming industries across the board. Let’s look at some examples:
1. Automotive Manufacturing
A major car manufacturer reduced downtime by 30% after implementing AI-driven predictive maintenance. Sensors tracked equipment conditions, and AI alerted staff about potential malfunctions days in advance.
2. Food and Beverage
AI ensured compliance with hygiene and safety standards by monitoring critical equipment. Predictive insights helped avoid spoilage by maintaining optimal conditions.
3. Energy Sector
Wind turbines equipped with IoT sensors and AI monitoring extended the lifespan of components, saving millions in repair costs annually.
Challenges and Limitations
While AI-driven predictive maintenance offers tremendous benefits, implementing it is not without challenges:
- Data Quality: Poor-quality or insufficient data can lead to inaccurate predictions.
- Integration Issues: Integrating AI systems with legacy infrastructure can be complex.
- Cost of Implementation: High initial investment may deter some businesses.
- Skill Gaps: Organizations need trained personnel to manage and interpret predictive systems.
The Future of Predictive Maintenance
As manufacturing processes become increasingly digital, the future of predictive maintenance lies in advanced technologies and innovative approaches. Let’s explore the trends shaping the next phase of this transformation.
Advancements in AI and Machine Learning
Self-learning algorithms are becoming more sophisticated, enabling them to adapt to new patterns and predict anomalies with greater accuracy. For instance:
- Reinforcement Learning: AI systems continuously learn from real-world actions, improving their predictions over time.
- Federated Learning: Distributed AI systems ensure data privacy by analyzing data locally while sharing insights globally.
Edge Computing
Edge computing processes data at or near the source rather than relying on centralized systems. This reduces latency and ensures faster, real-time insights, which is critical for environments like manufacturing floors.
Predictive Maintenance as a Service (PMaaS)
Cloud-based predictive maintenance solutions are emerging, allowing companies to adopt AI-driven systems without significant upfront costs. PMaaS offers:
- Scalability: Easy to expand across multiple facilities.
- Flexibility: Subscription models that adapt to business needs.
Integration with Digital Twins
A digital twin is a virtual replica of a physical asset. By integrating predictive maintenance with digital twins, manufacturers can simulate various scenarios to understand potential failures and refine their maintenance strategies.
Getting Started with AI-Driven Predictive Maintenance
Implementing predictive maintenance requires a structured approach to maximize its benefits. Here’s a roadmap to get started:
1. Assess Your Needs
Identify critical assets where failures could cause the most disruption. Evaluate your current maintenance processes to pinpoint areas for improvement.
2. Invest in IoT and Data Collection
Install IoT sensors to collect data on critical parameters like vibration, temperature, and pressure. Ensure your data sources are reliable and comprehensive.
3. Choose the Right Platform
Select a predictive maintenance solution that aligns with your business needs. Key features to look for include:
- Scalability for future growth.
- Integration capabilities with existing systems.
- User-friendly interfaces for easy adoption.
4. Train Your Team
Provide training for technicians and operators to interpret AI-generated insights. Upskilling your workforce ensures smoother integration and long-term success.
5. Monitor and Optimize
Track the performance of your predictive maintenance system using KPIs such as:
- Reduction in unplanned downtime.
- Cost savings on maintenance.
- Increased equipment lifespan.
Overcoming Challenges
Despite its advantages, AI-driven predictive maintenance comes with implementation challenges. Here’s how to address them:
- Data Quality Issues: Use advanced data cleaning tools and techniques to improve the accuracy of your predictions.
- Integration with Legacy Systems: Work with experienced vendors to ensure seamless integration with existing infrastructure.
- Skill Gaps: Partner with training providers to upskill your team in managing AI and IoT technologies.
- Cost Concerns: Start small with pilot programs to demonstrate ROI before scaling up.
Realizing the Benefits: A Case Study
Industry: Automotive
Challenge: High downtime due to unexpected failures in assembly line robots.
Solution: The company installed IoT sensors on critical components and used an AI-driven predictive maintenance platform.
Results:
- 30% reduction in downtime.
- 20% increase in overall equipment effectiveness (OEE).
- ROI achieved within six months.
This success story highlights the tangible benefits of predictive maintenance when implemented correctly.
The Broader Impact
AI-driven predictive maintenance doesn’t just save money—it transforms how manufacturing operates:
- Customer Satisfaction: By avoiding production delays, companies can meet delivery deadlines consistently.
- Environmental Benefits: Predictive maintenance reduces energy consumption and material wastage, supporting sustainability goals.
- Competitive Edge: Early adopters of AI-driven systems position themselves as industry leaders, capable of higher efficiency and reliability.
Conclusion
AI-driven predictive maintenance is reshaping the manufacturing landscape by making operations more efficient, cost-effective, and reliable. With advancements in AI, IoT, and edge computing, the potential for further transformation is immense.
TLDR
AI-driven predictive maintenance leverages machine learning, IoT, and data analytics to predict and prevent equipment failures in manufacturing. It enhances operational efficiency, reduces downtime, and ensures safety while providing significant cost savings.
FAQs
Predictive maintenance is a proactive approach to maintenance that uses data and advanced analytics to predict equipment failures before they occur. This helps reduce downtime and maintenance costs.
AI enhances predictive maintenance by analyzing large datasets, identifying patterns, and predicting failures with high accuracy. Technologies like machine learning, IoT, and computer vision enable more efficient and timely maintenance actions.
Predictive maintenance is used across industries such as manufacturing, automotive, energy, aerospace, and pharmaceuticals. It helps these sectors reduce costs, improve efficiency, and ensure safety compliance.
Challenges include the need for high-quality data, integration with legacy systems, initial investment costs, and training personnel to use AI-driven tools effectively.
Begin by identifying critical assets, investing in IoT sensors and analytics platforms, and setting clear goals for predictive maintenance outcomes. Partnering with experienced vendors can also streamline the implementation process.
Work with us