Support center +91 97257 89197
Generative AI developmentJuly 30, 2024
How Generative AI is Enhancing Diagnostics and Patient Care
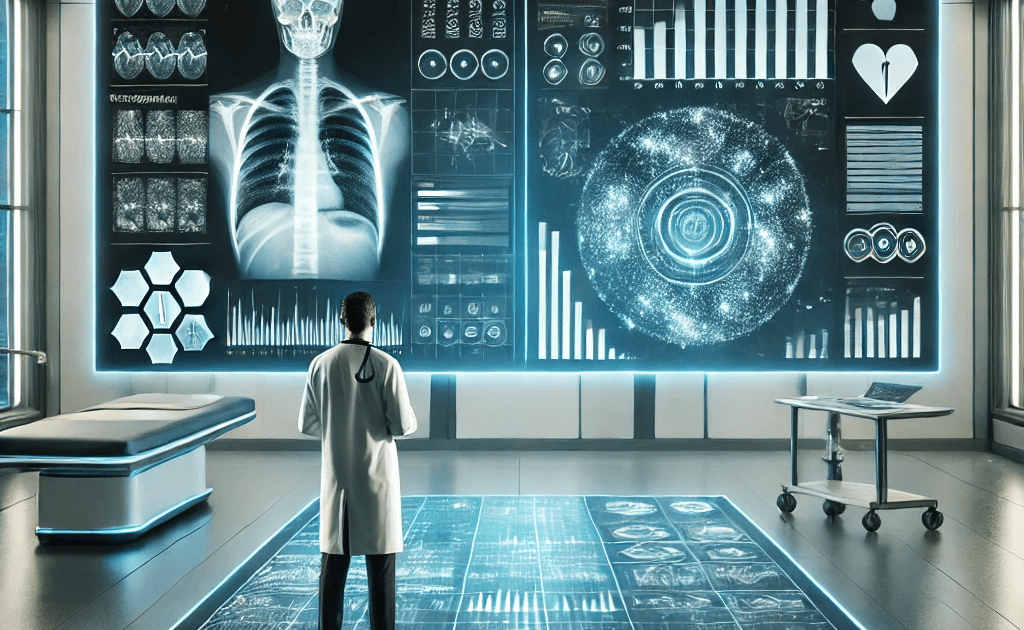
Introduction
In recent years, Generative AI has gained significant traction across various industries, with healthcare being one of the most promising fields. From early detection of diseases to enhancing personalized care, this innovative technology is revolutionizing the way we approach medical diagnostics and patient treatment. The power of generative AI lies in its ability to generate new data insights and solutions by leveraging deep learning and neural networks, a step beyond traditional AI applications in healthcare.
This article explores how generative AI is transforming diagnostics and patient care, highlighting its key applications in early disease detection, imaging, drug discovery, and personalized treatment plans. As healthcare systems across the globe face increasing demands for accuracy and efficiency, generative AI emerges as a critical tool for driving these advancements.
Understanding Generative AI and Its Role in Healthcare
Generative AI is a subset of artificial intelligence that can generate new content, data, or predictions based on existing datasets. It operates using advanced models like Generative Adversarial Networks (GANs) and Variational Autoencoders (VAEs), which allow it to create new outputs, such as diagnostic images or personalized treatment suggestions. In healthcare, this is a game-changer, as it can analyze complex medical datasets to provide insights that may not be immediately obvious to human doctors.
Before the advent of generative AI, healthcare already benefited from AI technologies, particularly in areas like medical imaging, predictive analytics, and robot-assisted surgery. However, these were largely reactive, depending on pre-defined rules or pre-existing data patterns. Generative AI moves beyond these constraints by actively generating new solutions, making it particularly useful for diagnosing rare diseases, personalizing treatments, and even accelerating drug discovery.
For example, while traditional AI might analyze an X-ray and identify potential issues based on pre-trained data, generative AI could create a more nuanced model, predicting disease progression or even generating future imaging that can forecast a patient’s health outcomes. This represents a leap forward in the accuracy, speed, and personalization of medical care.
Generative AI in Diagnostics
Early Detection and Predictive Analytics
One of the most critical aspects of healthcare is early disease detection, which can significantly improve patient outcomes, especially in life-threatening conditions like cancer and cardiovascular diseases. Generative AI’s predictive models analyze vast amounts of patient data—from genetic information to lifestyle factors—and identify patterns that might signal the early stages of a disease.
For example, in cancer detection, generative AI tools can review historical medical images and generate predictive insights, flagging patients who may develop cancer long before traditional diagnostics can detect it. By using patient history, family genetics, and lifestyle data, AI models can predict the likelihood of developing chronic diseases, allowing for timely interventions that are customized to the individual.
This level of precision in predictive analytics helps shift the healthcare focus from reactive to preventive care. With generative AI, healthcare providers can proactively identify at-risk patients and take steps to mitigate potential health issues before they become serious.
Imaging and Radiology
Medical imaging is another area where generative AI is making significant strides. Traditional diagnostic methods such as X-rays, MRIs, and CT scans have long been essential tools in diagnosing diseases. However, these tools often rely on human interpretation, which can be limited by time, human error, or the sheer volume of images that need analysis. Generative AI can assist radiologists by rapidly analyzing large datasets of medical images, identifying abnormalities, and even generating new insights based on the data.
One particularly exciting development in this field is AI-assisted anomaly detection. In some cases, generative AI has been able to detect minor anomalies in imaging data that were previously missed by human experts. For example, AI algorithms have been trained to identify early signs of diseases like lung cancer or Alzheimer’s from medical scans with remarkable accuracy.
Generative AI also helps reduce diagnostic errors and improves the speed of decision-making. In emergencies, where quick diagnostic turnarounds are crucial, AI-driven imaging can offer life-saving efficiency by delivering near-instant results with high accuracy.
Precision Medicine and Personalized Treatment Plans
A core strength of generative AI is its ability to enable precision medicine—an approach where treatments are customized to fit the individual characteristics of each patient. Generative AI can analyze a patient’s genetic makeup, medical history, and current health status to generate a personalized treatment plan. This level of care goes beyond the one-size-fits-all approach that traditional medicine often relies on.
For example, in treating diseases like diabetes or hypertension, generative AI can help doctors determine which medications will be most effective based on the patient’s unique biology. It can even recommend lifestyle changes that complement medical treatment, offering a holistic approach to health.
AI-generated personalized care plans can also help reduce unnecessary treatments and prevent adverse reactions to medications. For instance, if a patient is genetically predisposed to react poorly to a certain drug, generative AI can flag this in advance and suggest alternatives, making treatment safer and more effective.
Improving Patient Care with Generative AI
Virtual Health Assistants
Beyond diagnostics, generative AI is transforming the patient care experience through virtual health assistants. These AI-driven tools can communicate directly with patients to provide guidance, answer questions, and even manage appointments. Virtual assistants are available 24/7, offering patients immediate responses to their healthcare concerns without the need to visit a doctor’s office.
These AI tools also help patients manage chronic conditions by tracking their symptoms, sending medication reminders, and offering real-time advice on managing their health. For healthcare providers, this means reduced workload, allowing doctors to focus on more complex cases while AI handles routine inquiries.
Furthermore, virtual health assistants enhance patient engagement by ensuring that patients have a reliable and accessible source of information, improving compliance with treatment plans and increasing patient satisfaction.
Personalized Patient Experience
Generative AI also plays a critical role in delivering a personalized patient experience, particularly in areas like mental health and rehabilitation. By analyzing patient data, including emotional responses and progress over time, AI tools can customize care strategies, ensuring that each patient receives treatment tailored to their unique needs.
For example, in mental health, generative AI can create personalized therapeutic models based on patient responses, continuously adapting to ensure the most effective interventions. In rehabilitation, AI tools can monitor a patient’s progress in real-time, offering feedback and suggestions for improvement, making the recovery process more interactive and effective.
Generative AI also contributes to continuous monitoring. Wearable health devices, powered by AI, enable doctors to monitor patients in real-time, sending alerts for any concerning trends in vital signs or patient-reported symptoms. This real-time data can be used to adjust treatment plans on the fly, ensuring the best possible outcomes for the patient.
Drug Discovery and Development
One of the most transformative applications of generative AI in healthcare is its role in drug discovery and development. Traditionally, developing new drugs can take years, if not decades, with immense costs and rigorous trial processes. Generative AI is helping to accelerate this process by analyzing vast datasets of molecular structures and predicting how different compounds will interact with biological targets.
For example, AI models like Generative Adversarial Networks (GANs) can generate potential drug candidates by learning from existing molecular patterns and simulating interactions with human proteins. This speeds up the identification of compounds that have a high likelihood of success in clinical trials. AI can also help in repurposing existing drugs for new treatments by generating hypotheses on how they might interact with different diseases, as was seen during the rapid drug development efforts for COVID-19.
Generative AI can streamline clinical trials by predicting which patients are most likely to benefit from a new drug, allowing for more targeted trial designs. This results in faster trials, reduced costs, and potentially quicker approvals from regulatory bodies.
An example of this is the collaboration between Insilico Medicine and several pharmaceutical companies, where generative AI identified new drug candidates within months—far shorter than the traditional drug discovery timeline. This demonstrates AI’s potential to revolutionize not only the discovery process but also how medications are tested and brought to market.
Ethical and Practical Considerations
While the potential for generative AI in healthcare is immense, it is not without challenges. As with any powerful technology, there are important ethical and practical considerations to address.
Data Privacy and Security
Generative AI relies on access to massive amounts of healthcare data, including sensitive patient information. While this data is crucial for training AI models, it raises significant concerns about privacy and data security. Ensuring that patient data is protected, anonymized, and only used for legitimate purposes is essential. Strict HIPAA compliance and other regulatory frameworks must be adhered to, particularly as AI becomes more integrated into healthcare workflows.
In addition, hospitals and healthcare institutions need to invest in robust cybersecurity measures to prevent data breaches. Given the value of healthcare data, it is an attractive target for cybercriminals. If AI systems are compromised, it could have devastating effects on patient trust and healthcare operations.
AI Bias and Fairness
Another concern is the potential for bias in AI models. If generative AI is trained on datasets that do not adequately represent diverse populations, there is a risk that the AI’s recommendations could be skewed, leading to unfair or inaccurate outcomes for certain patient groups. For example, an AI model trained primarily on data from Caucasian patients might underperform in diagnosing diseases in patients of other ethnicities, exacerbating existing health disparities.
To mitigate this, healthcare organizations must ensure that AI models are trained on diverse datasets that represent all patient demographics. In addition, regular auditing of AI systems for bias should be a standard practice, ensuring that the AI’s decisions are fair, accurate, and inclusive.
Over-Reliance on AI
As AI systems become more advanced, there is a risk of over-reliance on these tools, leading to reduced human oversight in critical decision-making processes. While generative AI can assist in making more informed diagnoses and treatment decisions, healthcare providers must remember that AI should complement, not replace, human expertise.
Healthcare professionals still play a vital role in interpreting AI-generated insights and making final decisions based on their knowledge and experience. Maintaining a balance between AI-driven recommendations and human judgment is crucial to ensuring high-quality patient care.
Future Outlook
The future of generative AI in healthcare is incredibly promising, with numerous emerging technologies poised to further enhance diagnostics and patient care. As AI systems become more sophisticated, we can expect greater accuracy in diagnosis, faster drug discoveries, and even more personalized treatment plans.
Integration with Wearable Technology
One exciting area of growth is the integration of generative AI with wearable devices. Today’s wearables, such as smartwatches and fitness trackers, collect a wealth of health data from users, including heart rate, blood oxygen levels, and sleep patterns. Generative AI can analyze this real-time data to detect anomalies and predict potential health issues, allowing for earlier interventions.
For instance, a wearable device powered by AI could predict an impending heart attack based on subtle changes in heart rate variability, giving the user and their healthcare provider the ability to take preventive action. As wearable technology continues to advance, AI could become a crucial tool in managing chronic diseases and preventing acute medical events.
AI-Assisted Surgery and Robotics
In the realm of surgery, generative AI is expected to play a more prominent role in robot-assisted surgeries. By analyzing patient data and surgical outcomes, AI can assist surgeons in planning and executing procedures with higher precision. This reduces the risk of human error and improves patient outcomes.
For example, AI-assisted robotic systems could use patient data to generate a detailed plan for surgery, anticipating potential complications and adjusting the procedure in real-time based on the patient’s condition. As this technology evolves, we may see a future where AI plays a key role in performing complex surgeries with minimal human intervention.
AI-Driven Mental Health Support
Another area where generative AI is expected to make a significant impact is in mental health care. AI-powered tools can offer personalized mental health support by analyzing patterns in a patient’s communication, behavior, and biometric data. For example, AI-driven chatbots could provide therapeutic interventions tailored to the user’s needs, offering real-time support and even generating customized mindfulness exercises or cognitive behavioral therapy techniques.
This type of AI-driven support can complement traditional therapy, making mental health care more accessible, especially for those who may not have regular access to a therapist. As AI models become more adept at understanding human emotions and mental states, we could see a new era of personalized, always-available mental health care.
Conclusion
Generative AI is undeniably enhancing the landscape of healthcare, particularly in diagnostics and patient care. From early disease detection and personalized treatment plans to accelerating drug discovery and creating more responsive patient care systems, the applications of this technology are vast and transformative.
However, with this innovation comes the need to address important ethical considerations, such as data privacy, AI bias, and the role of human oversight in AI-driven systems. As healthcare continues to evolve with AI integration, it is crucial to strike a balance between embracing cutting-edge technologies and maintaining the trust, safety, and fairness that are foundational to patient care.
In the future, generative AI will likely become an even more integral part of healthcare, helping to achieve outcomes that were once thought impossible. As we continue to explore new frontiers in AI and healthcare, we are moving toward a world where medical treatment is faster, more accurate, and uniquely tailored to every individual.
TLDR
Generative AI is transforming healthcare, particularly in diagnostics and patient care. It aids in early disease detection, creates personalized treatment plans, and improves patient engagement through AI-driven tools. However, ethical concerns like data privacy and fairness must be addressed. This article explores how generative AI is being used today and its potential for revolutionizing healthcare in the future.
FAQs
Generative AI in healthcare refers to AI systems that use deep learning to generate data-driven insights, including diagnostics, treatment plans, and patient care strategies. It differs from traditional AI by its ability to create new solutions rather than just analyzing existing data.
Generative AI enhances diagnostics by analyzing complex datasets like medical images, lab results, and patient history to detect diseases early, provide predictive analytics, and recommend personalized treatments that may be more accurate than traditional methods.
Yes, generative AI helps personalize patient care by analyzing individual health data to tailor treatments, predict disease progression, and provide virtual assistants for real-time patient support, improving overall healthcare outcomes.
There are several ethical concerns, including data privacy, AI bias, and the risk of over-reliance on AI tools without human oversight. It’s essential to balance technological advancements with stringent data protection policies and human involvement.
The future of generative AI in patient care includes more advanced predictive analytics, integration with wearable technology for continuous health monitoring, and its role in accelerating drug discovery and personalized medicine tailored to each patient’s genetic profile.
Work with us